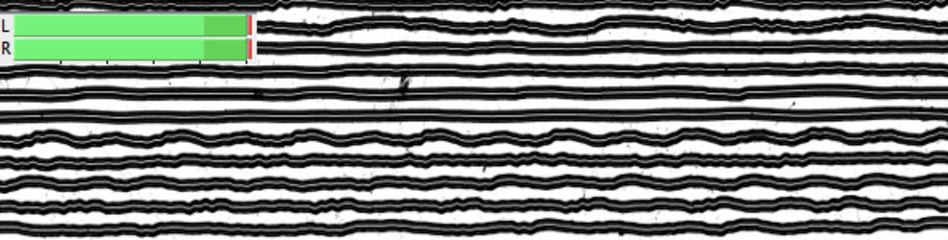
Introducing simple open-source tools for performative speech analysis: Gentle and Drift
Marit MacArthur

When we listen to a poetry reading — recorded or live — we constantly, half-consciously assess how well the poet captures and keeps our attention. I do not need to tell poets, and those who study poetry, that the words of a poem are only half of the equation, sometimes less. Pitch and pitch range, intonation patterns, volume/intensity, speaking rate/tempo, rhythm, stress/emphasis, vocal timbre — such paralinguistic features affect our experience and interpretation of a performed poem. I say performed, rather than read, because every poetry reading is a performance — even if Poets & Writers’ Funding for Readings & Workshops application would have us think otherwise:
Among paralinguistic features, intonation patterns — the rise and fall of vocal pitch — interest poets a great deal. The poetics of Robert Frost, for one, hinge on the “tone of meaning … without the words” (“Never Again Would Bird’s Song Be the Same”). In research on the perception of tone, Jody Kreiman and Diana Sidtis note:
Some authors … have claimed that normal adults usually believe the tone of voice rather than the words. Experimental studies suggest that … this … depends on how large the discrepancy is between the emotional and the linguistic meaning and how context is guiding the listener’s perceptions. For example, the contrast in “I feel just fine” spoken on a tense, tentative tone might be politely ignored, while, “I’m not angry” spoken in “hot anger” would not. Extreme discrepancies between the semantics and the emotional prosody stand out as anomalous … We notice when inconsistencies (which are the basis of verbal irony and sarcasm) occur, and often these incidents incite perplexity, fear, or humor (305, 304)
Poetry performance is full of such anomalies — in which the tone of voice contrasts with the emotional content of a poem, in part because of a default neutral style of academic poetry reading. While tone cannot be reduced to intonation patterns, “the fundamental frequency of the human voice [pitch] … heads the list of important cues for emotional meanings” (311).
After a reading, we are often quick to generalize: That was an impressive performance! Or: Wow, that put me to sleep. Or: Meh. We share similar impressions of political speeches, sermons, lectures, homilies, stand-up comedy routines, and so on. And to each instance of performative speech, we bring expectations that have been shown to color and alter our auditory perceptions. Such expectations arise from our own gender and cultural identifications, affiliations and listening experiences, and from assumptions we make about the speaker’s gender, sexuality, ethnicity, age, class, education, religious affiliation, historical period, geographic region, not to mention the reading venue, audience, recording format, etc.
In a recent essay in PMLA, I began to trace the evolution of poetry reading styles in the twentieth and twenty-first centuries, and locate the origins of the default, neutral style of contemporary academic poetry readings in secular performance and religious ritual. I used line graphs of intonation patterns (pitch contours) to visualize distinct performance styles such as monotonous incantation, popularly known as “poet voice,” which is characterized by: (1) the repetition of a falling cadence within a narrow range of pitch; (2) a flattened affect that suppresses idiosyncratic expression of subject matter in favor of a restrained, earnest tone; and (3) the subordination of conventional intonation patterns dictated by syntax, and of the poetic effects of line length and line breaks, to the prevailing cadence.
Since I finished that piece, I have begun to analyze additional paralinguistic features of poetry readings. My efforts have been supported in 2015–16 by an ACLS Digital Innovations Fellowship, which has allowed me to collaborate with a linguist specializing in phonetics, Georgia Zellou, at the University of California, Davis, as well as experts in the fields of interface design, audio signal processing, and machine learning and the neurobiology of auditory perception. One of my projects with Zellou compares a corpus of conversational speech and read speech with recordings by 60 poets, drawn from PennSound, the Academy of American Poets, and other online archives. The aim is to employ computational and statistical methods and machine learning to build on nuanced insights of close listening, moving beyond categorization of poets according to so-called neutral and dramatic styles, or according to poetic movement, school or clique. A second project compares the poetry reading styles of undergraduates not majoring in creative writing with the reading styles of graduate students in creative writing and professional poets.
Here I want to make several assertions that, I hope, others can prove wrong, or at least challenge with more counterexamples. My sense is that the tools commonly used in linguistics to analyze paralinguistic features of speech — even in sociolinguistics, psychoacoustics, sociophonetics, and the study of intonation — have rarely been applied to performative speech, such as poetry readings, political speeches, talking books, sermons, stand-up comedy, theatre and film acting, etc. (Rosario Signorello’s recent work on charisma in political speeches is one happy exception. Another early linguistic project is the Speech Lab Recordings, which Chris Mustazza is researching, and which began recording major poets at Columbia University in 1931.) Indeed, the scientific study of the physical properties of speech production, and the neurobiology of speech perception, are relatively young areas of research within the fields of linguistics and neuroscience. And as a rule, linguists tend to focus their research on so-called natural speech, recorded in sound labs. And so, as I begin to try to quantify vocal styles in performative speech, I am both excited and agnostic about what I may discover.
One of the primary goals of my research in 2015–16 has been to help develop and provide access to simple, open-source, user-friendly tools for humanistic research on vocal recordings, tools that work well on the noisy, low-quality recordings often found in the audio archive. My hope is that such tools can be used by humanistic scholars to refine close listening methodologies — in part by testing our deep and nuanced cultural knowledge about individual recordings and literary and cultural history, and dominant narratives about trends in vocal performance, against quantitative data about the paralinguistic features in a given vocal recording. A long-term ambition is to use machine learning to test assumptions about vocal performance styles and use supervised learning to explore large archives like PennSound. This project builds on the important work of Charles Bernstein, Al Fireis, Kenneth Sherwood, Chris Mustazza, Steve McLaughlin, Steve Evans, David Tcheng, David Enstrom, Tony Borries, Loretta Auvil, and others with whom I have worked as a participant in the NEH-sponsored High Performance Sound Technologies for Access and Scholarship (HiPSTAS) project, directed by Tanya Clement.
As Clement, Mustazza, and others have noted, quantitative, machine-assisted vocal analysis tools are crucial to research on audio because of the overwhelming size of some audio archives (see Clement) — and, I would add, because auditory perception is highly subjective. A notable linguistics experiment demonstrated that undergraduates perceive the exact same recorded lecture to be more difficult to understand if they are shown a photograph of an Asian-looking lecturer, and told that that person gave the lecture, rather than a photograph of a Caucasian-looking lecturer (cited in Eidsheim; see Rubin). The McGurk effect refers to the fact that we take visual (lip-reading) information into account even when it contradicts auditory speech perception (see Sekiyama). Researchers have also begun to investigate the ways that perceived gender influences speech perception (see Strand, Junger et al). Nevertheless, many of us “view [our] senses as documentary devices that faithfully translate the environment into understandable and manageable units … [we] accept what they see and hear” (von Hippel, Sekaquaptewa, and Vargas, 181). Such research suggests that vocal analysis tools using machine learning may serve as a corrective to unspoken assumptions about the vocal performance styles of individual poets, politicians, religious figures, radio and television commentators, and comedians, among others. Given the overwhelming number of recordings in many audio archives, machine learning may also help us look for and find patterns beyond the small group of recordings we get to know intimately.
***
Without further ado, I would like to introduce two new vocal analysis tools, Gentle, which I had the good fortune to discover, and Drift, whose development I have been able to support.
Gentle, developed in 2015 by Robert Ochshorn and Max Hawkins, is a powerful forced aligner that lines up a given transcript with an audio recording, word by word. It is built on top of an open-source speech recognition toolkit developed at Johns Hopkins University, Kaldi, which uses modern neural network-based acoustic modeling. (Ochshorn, it is worth mentioning, works for the Communications Design Group (CDG) Labs, founded in 2014 by Alan Kay and Bret Victor on the model of Xerox PARC; Hawkins, a 2013 graduate from Carnegie Mellon University in computer science and art, has already put in time on Google’s Data Arts Team. I am grateful to Dave Cerf for introducing me to them and their work.) Gentle was designed specifically to function with more flexibility than FAVE (Forced Alignment and Vowel Extraction), a tool developed in the Linguistics Lab at the University of Pennsylvania and commonly used by linguists, to be “easier to install and use … handle noisy and complicated audio … and … be unusually accommodating to long or incomplete transcripts.” Gentle also works well with some musical recordings, particularly hip-hop and rap.
Here is a screenshot of Gentle’s current interface online:
A user simply uploads an audio file, with or without a transcript, and clicks “Align.” After a few seconds or a minute or so (depending on the length of the recording), Gentle produces a playable transcript of the audio file, with options to download the transcript with precise timing information as a CSV or JSON.
The screenshot here shows Gentle’s playable transcript for Harryette Mullen’s “Present Tense,” followed by the beginning of the CSV. (Words in gray, in this case “divorce,” indicate that Gentle did not recognize the word in this particular recording.)
The data in the CSV, which includes each word’s duration as well as the length of pauses between words, can be used to calculate the speaking rate/tempo, the degree of regularity of the rate/tempo, and the rhythm, as well as to investigate how much a speaker pauses at the end of line, sentence or phrase, or between stanzas.
Gentle can also produce a rough transcript of a vocal recording from scratch, which can then be corrected and aligned with the recording. This feature has great advantages in research on poetry recordings and other audio common in humanistic research, as transcripts for many recordings do not exist or are not easily accessible, in part because of copyright law. Here is a screenshot of a playable rough transcript of “Present Tense,” which Gentle produced without the text of the poem:
The mistakes are amusing in many cases — and I, for one, would much rather begin with a rough transcript that I can create than find electronic versions of the text of every copyrighted poem I might want to analyze.
Drift, the second tool, is a highly accurate pitch-tracker that also incorporates the forced alignment features of Gentle, visualizing a pitch trace over time and aligning it with a transcript. Drift was prototyped in 2016 by Hawkins and Ochshorn, with funds from my ACLS Digital Innovations Fellowship project budget. Using an algorithm developed by Byung Suk Lee and Daniel P. W. Ellis at Columbia University to work with precise accuracy on the noisy, low-quality vocal recordings common in the audio archive, Drift measures vocal pitch (the fundamental frequency, the vibration of the vocal cords, as measured in hertz) every 10 milliseconds in a given recording. (Dan Ellis was involved in the
Here is a screenshot of Drift’s current interface online:
Again, I’ve used Mullen’s “Present Tense” as an example, alongside William Butler Yeats reading “The Lake Isle of Innisfree,” for comparison’s sake, though I will not elaborate a comparison here. Once Drift has processed the uploaded audio file, the user clicks on the “Done” button to see the pitch trace and waveform in a new window.
The user then pastes a transcript into the box on the lower left and presses “Submit” to align them.
The downloadable CSV from Drift provides the following data, which can be used to calculate mean and median pitch, standard deviation from mean and median pitch, and, I hope, intonation patterns:
As yet, the transcript and word duration data do not appear in the same CSV as the pitch data, nor do the values for intensity. In the next phase of the project, they will be combined in a single downloadable CSV.
I want to emphasize that these open-source tools are live online, at http://lowerquality.com/gentle and http://lowerquality.com:9876/upload, ready to use, and the code is available on GitHub. Please try them out. (If users want to use them on a large scale, please use the code; Gentle can be installed now, and an easy install of Drift should be available within the next year. Please do not overwhelm the lowerquality server.) And please contact me about your results, and with your questions!
***
Two brief postscripts.
1. For the PMLA piece mentioned above, I used the program ARLO (Adaptive Recognition with Layered Optimization), whose development has been supported by HiPSTAS. I am grateful to David Tcheng and Tony Borries for their work on ARLO in general, and on pitch-tracking in particular. In the case of noisy recordings, ARLO and Drift both track pitch better than Praat, an open-source program commonly used by linguists that not user-friendly. With two undergraduate research assistants, Pavel Kuzkin and Daphne Liu, I am currently testing Drift and for the foreseeable future, I plan to use it for pitch-tracking data rather than ARLO. So far, Drift produces more complete pitch data with fewer errors than ARLO (octave jumps are the bane of pitch-tracking, for familiar reasons I will not go into here), without the need to adjust parameters for different types of recordings. In this interface for ARLO’s pitch-tracker, which Tony Borries developed in 2015 with funding from my ACLS Digital Innovations Fellowship, it is necessary to change the pitch range (“pitch trace start frequency” and “pitch trace end frequency”) for male or female speakers.
Researchers with access to ARLO who want to use it to track pitch will probably want to use the the settings in this screenshot for male voices, with the pitch start and end at 50–300 hz, and set the pitch start and end at 75–400 for female voices.
2. Christopher Grobe, a brilliant performance studies scholar and an English professor at Amherst College, has complained that the study of voice in poetry often “veers into dry dissection of the human vocal anatomy,” divorced from the body and larger cultural contexts (Grobe 217). This is a fair point. However, since I began research in 2012 on pitch-tracking and poetry performance, and especially since, at UC Davis, I audited Zellou’s graduate course in phonetics and have begun to collaborate a bit with Lee M. Miller, a neuroscientist who studies auditory and speech perception in noisy environments, I am in ever more awe of the human vocal anatomy and the complexity of auditory perception. I caution myself and other humanistic scholars about what we can say and how much we can understand about the voice, without acquiring further training, going back to graduate school in linguistics—and/or, which is more realistic, collaborating with others who have spent years studying vocal anatomy, speech production and auditory perception. The strength of humanistic scholars in such research is our deep cultural understanding of vocal performance, literary and cultural traditions. We have potent intuitions and rich questions. For technical methodologies, we must collaborate as much as we can. Now I must, ever so briefly, veer into dry dissection of the human vocal anatomy.
The seemingly indefinable characteristics of an individual voice depend, as I understand them, on the length of the vocal tract (which correlates with height), how open the voice box is (creaky voice or vocal fry results from a partially closed voice box), and all the idiosyncrasies of the interior of our throats and mouths (vocal timbre is influenced, among other things, by smoking and gastro-esophageal reflux disease) — not to mention, in the vocalization of a given utterance, the position of the tongue in relation to the teeth and the palate, the degree of nasality, and so on. And then there are all the influences of language and culture. The vocal tract of each human being is like a unique, intricate horn made of flesh and bone. No one has precisely the same instrument, and there are many ways to play it. And to listen.
Works Cited
Clement, T. “When Texts of Study Are Audio Files: Digital Tools for Sound Studies in DH.” A New Companion to Digital Humanities. Ed. Susan Schreibman, Ray Siemens and John Unsworth. New York: Blackwell, 2016: 348–357.
Eidsheim, Nina. “Marian Anderson and ‘Sonic Blackness’ in American Opera.” American Quarterly 63.3 (Sept. 2011): 1–19.
Grobe, Christopher. “The Breath of the Poem: Confessional Print/Performance Circa 1959.” PMLA 127.2 (March 2012): 215–230.
Junger, J. et al. “Sex Matters: Neural Correlates of Voice Gender Perception.” NeuroImage 79 (October 2013): 275–87.
Kreiman, Jody and Sidtis, Diane. Foundations of Voice Studies: An Interdisciplinary Approach to Voice Production and Perception. Wiley, 2011. Print.
MacArthur, Marit. “Monotony, the Churches of Poetry Reading, and Sound Studies.” PMLA 131.1 (January 2016).
Mullen, Harryette. “Present Tense.” Sleeping with the Dictionary. Berkeley: U of California P, 2002: 57.
———. “Present Tense (Audio Only).” Poetry.org. Audio. Acad. of Amer. Poets. July 20, 2001. Web. 5 April 2016.
Rubin, D.L. “Nonlanguage Factors Affecting Undergraduates’ Judgments of Nonnative English Speaking Teaching Assistants.” Research in Higher Education 33.4 (1992): 155–68.
Sekiyama, Kaoru. “Cultural and linguistic factors in audiovisual speech processing: The McGurk effect in Chinese subjects.” Perception and Psychophysics 59.1 (1997): 73–80.
Strand, Elizabeth. “Uncovering the Role of Gender Stereotypes in Speech Perception.” Journal of Language and Social Psychology 18.1 (March 1999): 86–99.
von Hippel, W., Sekaquaptewa, D., and Vargas, P. (1995). “On the Role of Encoding Processes in Stereotype Maintenance.” Advances in Experimental Social Psychology 27: (177–254).
Yeats, William Butler. “The Lake Isle of Innisfree.” Selected Poems and Two Plays of William Butler Yeats. New York: Macmillan, 1962: 12.
———. Oct. 28, 1936. PennSound. 15 April 2016.